Using the historical crime dataset and Machine Learning Models we have developed a crime prediction application to forecast where and when future crimes are likely to occur, law enforcement can then focus resources on these areas to deter crime. We are able to analyze patterns and trends in crime that may evade human analysis. There is continuous improvement in predictability as the data and models are refined. We considered two crime datasets of different cities, employing similar techniques for data preprocessing, splitting, feature selection, model training, and hyper-parameter tuning, with some variations in feature engineering specific to each dataset.
Results
Dataset 1
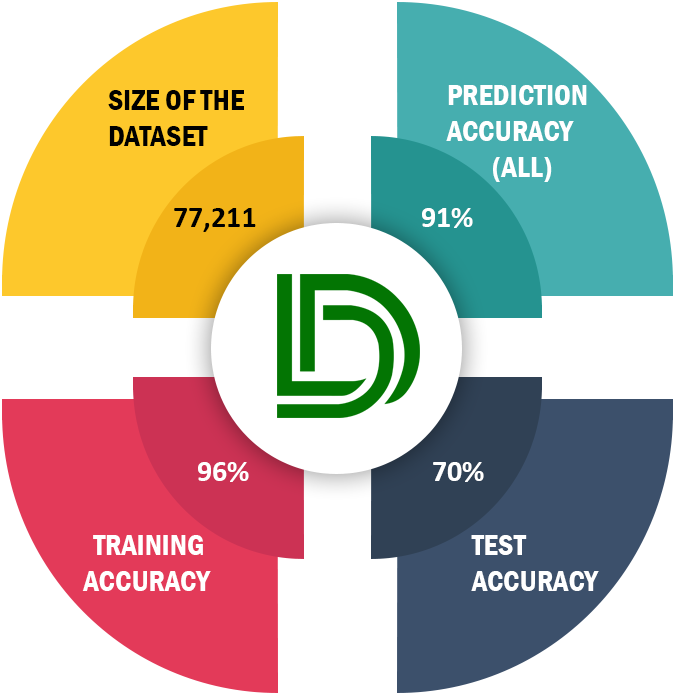
Dataset 2
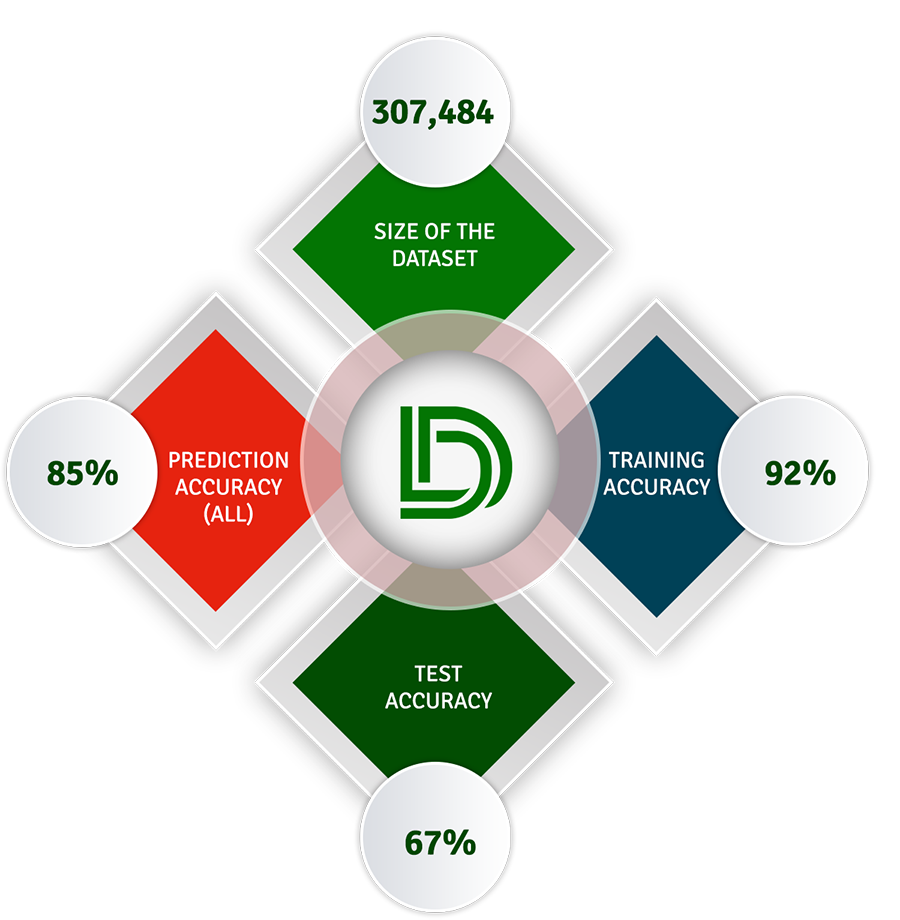
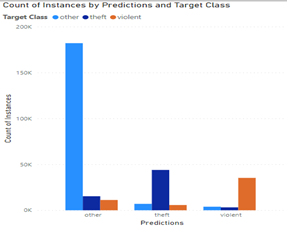
The two crime prediction datasets, Dataset 1 and Dataset 2, differ significantly in terms of model performance and feature composition. Dataset 1 exhibits higher training accuracy (96%) and superior generalization with a test accuracy of 70%, while Dataset 2 achieves 92% in training accuracy and 67% in test accuracy. Dataset 1 includes a wide range of demographic and temporal attributes, making it suitable for general crime prediction, while Dataset 2 emphasizes location-based features, making it more suitable for urban crime prediction. We enhanced our model's performance by engineering new features, tuning and optimizing hyperparameters, with Random Forest as the best-performing algorithm. This led to a 70% accuracy on unseen data in dataset 1, a significant improvement compared to the baseline of mathematically best 33% (randomly choosing from one of the three target variables - theft, violence or other).
In summary, this versatile solution is highly effective with other crime datasets, and a larger, richer dataset typically leads to improved model performance due to data and feature dependencies.
The flow diagram for the entire process: